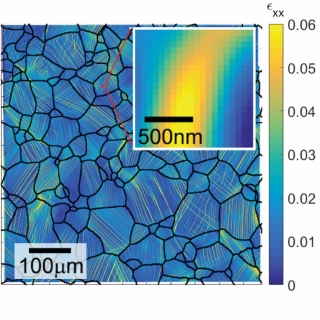
Data-Driven Approaches to Characterize Microstructure-Property Links
The emergence of new experimental capabilities for material characterization that result in extremely large and multi-dimensional data sets, combined with the concurrent emergence of unsupervised learning methods and computing power, has opened the door for a powerful means of unbiased recognition of the complex interactions between microstructure and mechanical properties under a wide range of thermo-mechanical loading conditions. The development of unsupervised learning frameworks has the potential to radically improve current abilities to predict the effects of processing and composition on material behavior, accelerating the development and deployment of new materials and increasing confidence in their reliability and performance. In view of this, our group works on the creation of experimental and analytical frameworks that will address length scales from the macroscale down to tens of nanometers, and that will be widely applicable to a broad range of materials and thermo-mechanical loading conditions. A combination of microscale deformation mapping (using distortion-corrected scanning electron microscopy to track and correlate chemically-functionalized nanoparticles on a specimen surface), electron backscatter diffraction for pre- and post- surface microstructural characterization, three-dimensional microstructural characterization, and globally-averaged macroscale material response are used to investigate the links between material microstructure and the initiation and evolution of microscale damage leading to material failure.